Abstract: In structural health monitoring, data quality is crucial to the performance of data-driven methods for structural damage identification, condition assessment, and safety warning. However, structural health monitoring systems often suffer from data imperfection, resulting in some entries being unusable in a data matrix. Discrete missing points are relatively easy to recover based on known adjacent points, whereas segments of continuous missing data are more common and also more challenging to recover in a practical scenario. Formulating the data recovery task as an optimization problem for matrix completion, we present a convolutional neural network to achieve simultaneous recovery for multi-channel data with the awareness of group sparsity. The data recovery process based on compressive sensing is formulated as a regression problem and achieved in the neural network. The basis matrix is utilized as the input and the incomplete data matrix as the output to provide partial information for approximation. Basis coefficient optimization is performed via convolutional operation. Group sparsity regularization is applied while updating the kernel of the convolutional layer. The recovery can be readily obtained after optimization (training) without further validation and testing. The proposed method does not need intact data prepared in advance for training; also, it can handle sporadic data loss and make the most of interrupted information. Recovery ability evaluations on synthetic data, field-test data, and monitoring data of seismic response indicate that the proposed method achieves a good recovery result with high loss ratio and continuous data loss. The code is available at https://github.com/dawnnao/Group-sparsity-aware-CNN.
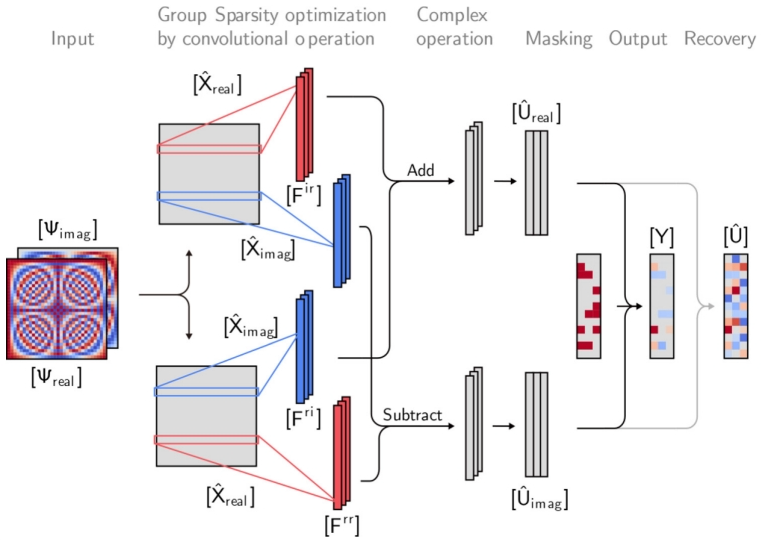